Imagine walking into a store where everything is tailored just for you. From the music to the app recommendations, it’s all about you. This isn’t magic. It’s machine learning, changing how brands reach out to customers.
At Netflix, this change saved over $1 billion by keeping viewers loyal. Blue Oak BBQ saw 28% of customers open emails, leading to 30% more dine-in sales. These stories show what machine learning can do when data and strategy come together.
Data-driven marketing is now essential. From Starbucks’ 38,000 stores to Devocion Coffee’s 15,000+ new customers yearly, leaders use AI to act on data. Machine learning algorithms find trends humans miss, predicting what customers want and keeping them from leaving.
Edley’s BBQ grew its subscriber list 20x in eight months. This shows even small teams can compete with big ones.
Key Takeaways
- Machine learning cuts costs and boosts revenue—Netflix saved $1B by retaining viewers.
- Blue Oak BBQ’s email campaigns achieved a 28% open rate, outperforming other channels by 30%.
- Generative AI could add $4.4 trillion to the global economy (McKinsey), making it a must for modern marketers.
- Tools like FedEx’s Nina resolve 80% of customer issues on first contact, proving AI’s impact on satisfaction.
- Accurate data and smart tools are critical: Sweet Wheat Bakery saved $10,000 quarterly using ML-driven analytics.
Understanding Machine Learning and Its Importance
Machine learning (ML) is a part of AI in digital marketing. It uses algorithms to learn from data and make decisions. This helps tools analyze customer behavior and predict trends, making optimizing marketing with machine learning key today.
What is Machine Learning?
Machine learning finds insights in data using statistical models. It’s different from static rules because it changes with new data. For instance, Netflix’s system suggests shows based on what you’ve watched, boosting user engagement by 40%.
How Machine Learning Works
- Data ingestion: Collecting information from websites, social media, and customer interactions
- Pattern recognition: Algorithms identify trends in data, like purchase habits or content preferences
- Model training: Systems refine predictions over time using historical data
Key Benefits for Marketers
“Marketers spend 16 hours weekly on repetitive tasks.” – HubSpot
ML automates these tasks, saving time and boosting efficiency. Here are the main benefits:
Benefit | Example | Impact |
---|---|---|
Data Analysis | Amazon’s product recommendations | 35% higher conversion rates |
Personalization | Spotify’s Discover Weekly | 25% increase in user retention |
Risk Reduction | PayPal’s fraud detection | 90% fewer fraudulent transactions |
Brands like Coca-Cola and Starbucks use ML to cut costs by 20–40% and improve targeting.
How Machine Learning Transforms Marketing Strategies
Artificial intelligence is now key in making marketing plans. It uses machine learning in marketing analysis to change how we talk to customers and work. This change helps marketing teams make decisions based on data, not just guesses.
Data-Driven Decision Making
Machine learning looks at huge amounts of data to find patterns we can’t see. For example, AI in email campaigns can guess what customers want, making them more likely to open emails. A 2023 study by Marketing Week showed that AI in email marketing can increase click-through rates by 22%.
Some key benefits are:
- Automated analysis of customer journey touchpoints
- Prediction of campaign performance using historical data
- Real-time adjustments to ad spend and messaging
Personalization and Customer Experience
Machine learning helps make experiences super personal. Think of Amazon’s recommendation engine, which is right 85% of the time. Now, websites can change what they show you based on how you act. This makes things better for customers.
Aspect | Traditional Marketing | Machine Learning |
---|---|---|
Recommendations | Static product lists | Behavior-based suggestions |
Segmentation | Demographic guesses | Behavioral micro-segments |
Response Time | Weekly/monthly adjustments | Real-time optimization |
“Customers today expect tailored experiences, and machine learning makes this scalable.” – Gartner Digital Marketing Report
These changes help save money on ads and make customers happier by 30% or more. By using AI, teams can spend more time on creative ideas and less on sorting through data.
Enhancing Customer Segmentation with Machine Learning
Modern marketing needs to segment customers well to boost engagement and sales. Old ways like demographics don’t fully get what customers do. Machine learning changes this by digging deep into big data to find patterns.
The Role of Algorithms in Segmentation
Algorithms like K-means, hierarchical clustering, and DBSCAN are key in today’s segmentation. They use data like what customers buy, how they browse, and how they interact to group them. For example:
- K-means groups customers based on what they do, helping personalize emails
- DBSCAN finds special groups in data that humans might miss
- Machine learning keeps these groups up-to-date as new data comes in
Predictive Analytics for Targeting
Predictive analytics goes further by guessing what customers will do next. It looks at past buying habits, cart abandonment, and how they respond to offers. This way, retailers can see a 20% better return on their marketing efforts. For instance, a fashion brand might send special deals to its most valuable customers while sharing tips with those who are less active.
Getting the data right is important—things like changing data types and removing odd values help. When done right, this can cut down on customer loss by 15% and increase loyalty by 60%. By mixing segmentation with live data, companies can make messages that feel made just for each person, turning insights into real plans.
Optimizing Marketing Campaigns with AI
Marketing strategies powered by artificial intelligence for marketing campaigns are changing how teams work. AI uses real-time data to adjust campaigns for better results. This means resources are used where they are most effective, boosting efficiency and growth.
Automating Campaign Adjustments
AI makes decisions that used to take a lot of manual work. For instance, it changes ad bids in real time to focus on the best channels. Tools like Google Ads Smart Bidding use machine learning to manage budgets and placements. Here’s how it works:
- Automated budget reallocation based on performance metrics
- Content A/B testing scaled across multiple platforms
- Dynamic ad creatives tailored to audience interactions
Real-Time Performance Monitoring
Artificial intelligence for marketing campaigns keeps an eye on metrics all the time, spotting issues right away. Platforms like HubSpot and Salesforce Marketing Cloud show dashboards for underperforming ads or customer groups. A 2023 study by Experian found that AI-driven email campaigns have 2.5× higher click-through rates than manual ones.
“Real-time insights let us pivot strategies mid-campaign, saving costs and boosting engagement.”
Regular optimization cycles keep campaigns on track with goals. Teams using automated marketing strategies cut wasted spend by up to 30%, a 2024 SurveyMonkey survey found. By focusing on big-picture decisions, marketers can grow ROI 40% faster. Keeping AI models updated with new data helps them keep up with changing consumer habits.
Leveraging Predictive Analytics in Marketing
Predictive analytics for marketing changes the game by moving from guesswork to foresight. It lets brands predict trends and what customers will do next. This way, businesses can plan better, use their money wisely, and get better results.
With the market growing fast, this tech is now a must-have for staying ahead. It’s not just a tool; it’s a key to success.
Approach | Traditional Marketing | Predictive Analytics |
---|---|---|
Decision Making | Based on past trends | Uses real-time data |
Customer Insights | Limited to historical data | Forecasts future behavior |
Resource Allocation | Guesswork | Data-driven optimization |
Anticipating Customer Behavior
Machine learning models help predict things like when customers might leave or what they might buy next. They use data to spot important customers. For instance, Coca-Cola got 25% better at promotions by using predictive analytics.
Apps can also tell when users might leave. This lets businesses reach out to them before they go.
Enhancing ROI with Predictions
Marketing that uses data can cut waste by 30% and keep customers longer by 15%. Netflix’s recommendations, for example, increased user engagement by 75%. This shows how well data-driven marketing works.
Marketers now spend money on channels that are likely to work. This makes sure every dollar counts. Tools like Tableau and Google Analytics 4 make it easier for everyone to use advanced analytics.
Data-driven strategies outperform intuition-based approaches by a 6-to 1 success ratio.
Brands that use predictive analytics today can see a 10-20% increase in sales. As privacy rules change, these tools help businesses grow while keeping customer trust.
Machine Learning Tools for Marketers
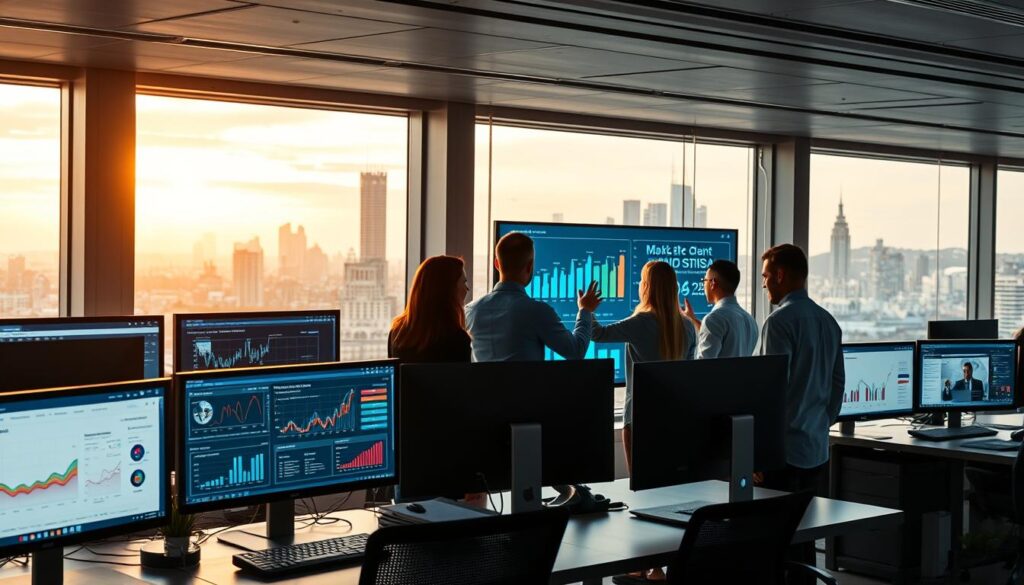
Choosing the right tools is key to unlocking the full marketing automation solutions can offer. Platforms like Crayon, Kompyte, and Brandwatch give you competitive intelligence. Drift and Enlyft make lead generation and qualification easier. MarketMuse and Surfer SEO use machine learning to improve content strategies, matching user intent and search trends.
- Crayon: Tracks competitor moves, classifying updates into 80+ categories.
- Kompyte: Generates sales-ready battlecards and win/loss analysis reports.
- Drift: AI chatbots qualify leads and recommend personalized product paths.
Integration is key. Use these tools with customer data platforms (CDPs) to unify datasets. This ensures optimizing marketing with machine learning fits with your existing tech stack. APIs make connecting to CRM systems like Salesforce easy. Platforms like NVIDIA’s H100 GPUs offer scalable computing power for complex analyses.
“AI tools cut manual work, letting teams focus on strategic growth.”
Devocion Coffee boosted engagement with Shopify and Klaviyo, showing how these tools can drive ROI. Over 45% of marketers say AI makes workflows simpler, according to DigitalOcean’s 2023 report. For more on balancing tech with mindful strategy, check out ZenJump’s resources on ethical implementation.
Begin by assessing tools based on your team’s size and goals. Look for platforms with clear ROI metrics, like MarketMuse’s content heatmaps or Surfer SEO’s keyword analysis. Make sure the tools you choose align with your long-term goals to avoid tech sprawl and maximize efficiency.
Case Studies: Successful Machine Learning Implementations
Machine learning has changed the game in marketing. It has helped top brands grow and become more efficient. Let’s look at how these technologies are making a difference.
Company | Challenge | Solution | Outcome |
---|---|---|---|
Netflix | Reducing churn and boosting retention | Recommendation engine analyzing viewing patterns | $1B annual savings from reduced customer attrition |
DoorDash | High marketing costs | Dynamic pricing and demand forecasting | 10-30% cost reduction without shrinking reach |
Starbucks | Personalizing promotions | Predictive models for local market insights | 20% increase in campaign ROI |
Spotify | Curating personalized playlists | Algorithmic music recommendations | 246M premium subscribers as of 2023 |
These stories highlight the power of machine learning in marketing. They teach us important lessons:
- Good data leads to better predictions (e.g., Netflix’s 80% recommendation-driven viewing)
- Testing and tweaking algorithms can greatly improve results (DoorDash adjusted weekly)
- Personalized content boosts engagement: Amazon’s recommendations make up 35% of its revenue
Even small businesses can use these tools. Start by finding machine learning solutions that match your goals. Remember, 56% of CMOs are investing in AI this year. It’s becoming a key part of modern marketing.
Overcoming Challenges in Machine Learning Adoption
Starting with machine learning comes with its own set of challenges. But, by tackling these, you can unlock the full AI in digital marketing power. Mid-sized businesses often face issues like talent shortages and budget constraints. But, there are ways to turn these obstacles into chances for growth.
IBM’s 2023 AI Adoption Index highlights five key obstacles: integration complexity, data fragmentation, high costs, skill shortages, and ethical risks.
Many mid-sized firms struggle with finding the right ML talent, with 71% citing this as a major issue. Another 29% face budget challenges. These hurdles can slow down automated marketing strategies. But, there are steps you can take to overcome them:
- Bridge skill gaps through partnerships with data science consultancies or upskilling teams via certification programs.
- Prioritize scalable solutions like automated data cleaning tools to reduce manual work.
- Start with pilot projects—like customer segmentation—to demonstrate ROI before full adoption.
Cost worries can be managed by focusing on the most impactful uses. For example, IBM’s framework suggests using a use-case catalog to focus on projects with clear benefits. Even smaller teams can start by automating simple tasks, which frees up time for more important work.
Being open and transparent is essential. Use ethical AI frameworks and talk openly with legal teams to handle compliance issues. Companies like Salesforce now offer pre-trained models that make ML more accessible, even with limited resources.
By facing these challenges head-on, businesses can stay ahead. Early adopters can see a 10-15% boost in customer segmentation alone. The journey starts small, but the benefits of AI in digital marketing adoption are significant and lasting.
Building a Machine Learning Team
Creating a machine learning team is key for using marketing automation solutions and learning data-driven marketing techniques. A good team mixes technical skills with marketing smarts. This way, they can make AI work for real results. With AI set to boost the global economy by $13 trillion by 2030, having the right team is vital to stay competitive.
Identifying Key Skills Required
Successful teams need three main skills:
- Technical skills: Knowing how to code, understanding data science, and being familiar with tools like Python or TensorFlow.
- Domain expertise: Knowing a lot about customer behavior, how to improve campaigns, and marketing automation solutions.
- Soft skills: Being able to communicate well, manage projects, and turn technical ideas into marketing plans.
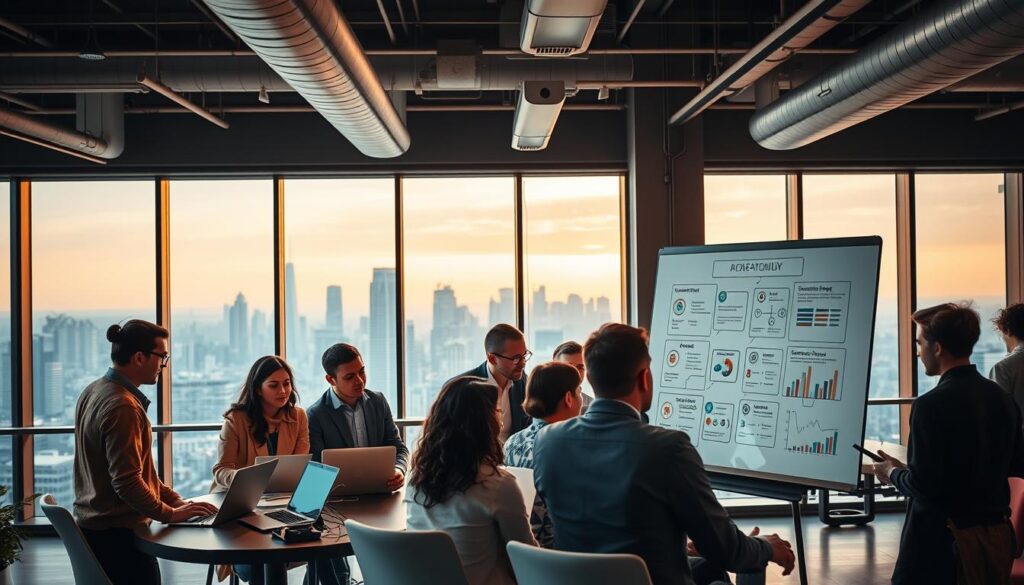
Collaborating with Data Scientists
Getting marketing and data science teams to work together needs a plan. Here are some strategies:
- Hold weekly meetings where both teams talk about goals and progress.
- Use the same KPIs like how accurate the models are and how much money campaigns make.
- Have workshops to show how data-driven marketing techniques help the brand.
Using data-driven marketing techniques means models help achieve business goals. Teams must also tackle issues like not talking enough and working in silos. By focusing on clear communication and learning from each other, teams can avoid problems and innovate.
Role | Core Responsibilities |
---|---|
Marketers | Strategy alignment, customer insights, campaign execution |
Data Scientists | Model development, data analysis, algorithm optimization |
IT/Engineers | System integration, tool deployment |
Future Trends in Machine Learning for Marketing
Artificial intelligence is changing marketing, and the future looks exciting. By 2024, 69.1% of marketers will use AI, with the market expected to hit $40 billion. This change is not just about new tools but also about new strategies. Emerging trends like autonomous systems and real-time optimization will change how teams work.
The Impact of AI on Marketing
Marketing teams will soon focus more on strategy and less on doing tasks. AI is now handling tasks like bid optimization and audience targeting. This lets humans focus on creativity and ethics.
For example, Google’s Smart Bidding uses machine learning to adjust campaigns in real time. But, there are challenges. 41% of CMOs are concerned about data privacy, and 60% fear AI could replace jobs. It’s important to find a balance between automation and human insight.
Emerging Technologies to Watch
- Natural language processing (NLP) will help create dynamic content, from ads to emails.
- Computer vision tools will analyze visual data to improve social media and product images.
- Federated learning will help create personalized campaigns without risking privacy.
Generative AI is making a big impact, with 42.2% of marketers seeing major changes from tools like DALL-E and GPT-4. Explainable AI is also important, as it helps build trust. Using these tools right means changing how we work, not just adding new tech. We need to keep training and setting ethical standards as we go.
Marketing with machine learning needs to be flexible. Marketers who are both tech-savvy and creative will lead the way. As AI takes over the details, humans will focus on guiding the direction and ensuring technology is used for good, not just for results.
Ethical Considerations in Machine Learning Marketing
As we use AI in digital marketing, we face big ethical challenges. We must balance new ideas with being responsible. This ensures leveraging machine learning in marketing analysis respects consumer rights and laws. We’ll look at how to meet these challenges without slowing down.
Data Privacy Concerns
Following GDPR and CCPA rules is a must. When we collect data for ML models, being open is key. Privacy-by-design helps us only collect what we really need.
Clear choices let users decide what to share or delete. Anonymizing data keeps identities safe while keeping analysis useful.
In a 2023 PwC survey, 81% of CMOs plan to adopt GenAI for new business models, underscoring the need for ethical guardrails now.
Building Trust with Consumers
Being open about how algorithms work builds trust. Telling customers how personalization works makes them feel more in charge. Regular checks for bias in data ensure fairness.
Guidelines for ethics help teams focus on fairness and accountability. When customers see their data handled well, they trust us more. This strengthens their loyalty to our brand.
Doing the right thing in AI marketing isn’t just about following rules. It’s a way to stand out. By checking our systems and valuing fairness, we make sure AI in digital marketing moves forward the right way.
Getting Started with Machine Learning in Marketing
Starting your machine learning journey involves clear steps and easy-to-use tools. Begin by matching your strategies with your business goals, like boosting lead conversion or cutting down customer loss. Marketing automation solutions like HubSpot or Google Analytics make it easier to get started. Data-driven marketing techniques help you track your progress.
Steps to Implement Machine Learning Strategies
First, check your data collection—customer interactions, CRM records, and social media insights—to find what’s missing. Start small by testing predictive analytics for campaign success or using chatbots for instant customer help. Tools like AWS Marketing AI or Salesforce Einstein help automate tasks. Use KPIs like CTR or conversion rates to improve your strategies over time.
Resources for Continuous Learning in AI
Look into certifications like Google’s AI Marketing Fundamentals or Coursera’s Machine Learning courses. Books like AI Marketing: An Advertising Revolution by Mike Bhargava connect theory to practice. Join groups like Kaggle or MarketingProfs to share knowledge. Focus on learning paths that include data-driven marketing techniques, like supervised learning models and segmentation tools.
Success comes from trying and improving. DoorDash cut costs by 20% with predictive analytics, showing small steps can add up. Be open to trying new things, from A/B testing to real-time bidding algorithms. Airbnb’s AI pricing shows how innovation can lead to market dominance. Stay flexible, focus on ethics, and let data lead your way to stay ahead in the AI world.
FAQ
What is the role of machine learning in modern marketing?
How does machine learning improve customer segmentation?
What are some benefits of using AI in digital marketing?
How do marketers use predictive analytics for marketing approaches?
What challenges do marketers face when adopting machine learning?
How can businesses ensure ethical use of machine learning in marketing?
What tools are available for marketers to leverage machine learning?
What should marketers consider when building a machine learning team?
What emerging trends should marketers look for in machine learning?
How can marketers get started with machine learning?
Source Links
- 10 Powerful ways to use data, machine learning and AI for marketing I GoGoGuest – https://gogoguest.com/blog/10-powerful-ways-to-use-data-machine-learning-and-ai-for-marketing
- AI in Marketing | IBM – https://www.ibm.com/think/topics/ai-in-marketing
- Unlock Profitable Growth: AI/ML Strategies for Revenue Optimization in Distribution – https://www.revologyanalytics.com/harness-ai-ml-in-distribution
- How Machine Learning Can Be Used in Marketing – https://mobidev.biz/blog/how-machine-learning-improves-marketing-strategies
- 10 Ways to Use Machine Learning for Marketing in 2025 – https://www.analyticsvidhya.com/blog/2023/03/machine-learning-for-marketing/
- Machine Learning in Marketing: A Guide (2024) – https://www.salesforce.com/marketing/ai/machine-learning/
- Finding the Mean Using Python – https://www.nobledesktop.com/learn/python/machine-learning-for-marketing-optimizing-campaigns-with-data
- How to Apply Machine Learning for Customer Segmentation – https://www.clicdata.com/blog/customer-segmentation-using-machine-learning/
- Customer Segmentation Models: Enhancing Targeted Marketing Strategies – https://www.rightpoint.com/thought/article/customer-segmentation-models-enhancing-targeted-marketing-strategies
- AI in Digital Marketing: Optimizing Campaigns – https://aiap.us/artificial-intelligence/ai-in-digital-marketing-optimizing-campaigns/
- How to use AI for marketing: A comprehensive guide – https://www.epsilon.com/us/insights/blog/how-to-use-ai-for-marketing
- Adjust’s guide to predictive analytics in mobile marketing | Adjust – https://www.adjust.com/resources/guides/predictive-analytics/
- Maximize Your Marketing ROI with Predictive Analytics – https://www.cardinalpath.com/blog/maximize-your-marketing-roi-with-predictive-analytics
- The Top 10 AI Tools for Marketers (Updated 2024) – https://www.pecan.ai/blog/top-10-ai-marketing-tools/
- 11 AI Marketing Tools to Accelerate Your Growth Strategy | DigitalOcean – https://www.digitalocean.com/resources/articles/ai-marketing-tools
- Machine Learning for Marketers – https://www.coursera.org/learn/machine-learning-marketing
- Best Examples of Machine Learning in Marketing – https://litslink.com/blog/best-examples-of-machine-learning-in-marketing-real-world-success-stories
- Successful AI Implementations in Marketing | DSM – https://digitalschoolofmarketing.co.za/digital-marketing-blog/case-studies-successful-ai-implementations-in-marketing/
- Top 30 Machine Learning Case Studies [2025] – https://digitaldefynd.com/IQ/machine-learning-case-studies/
- 5 Key Challenges Faced by Companies in Adopting Machine Learning – https://www.netguru.com/blog/challenges-in-adopting-machine-learning
- How to clear 5 hurdles to AI adoption in marketing analytics | MarTech – https://martech.org/how-to-clear-5-hurdles-to-ai-adoption-in-marketing-analytics/
- How to Build Machine Learning Teams That Deliver – https://neptune.ai/blog/how-to-build-machine-learning-teams-that-deliver
- What to Know to Build an Effective Marketing AI Strategy – https://consultport.com/business-excellence/what-to-know-to-build-a-marketing-ai-strategy/
- 10 Machine Learning Team OKR examples with initiatives – https://www.tability.io/templates/tags/machine-learning-team
- Leveraging AI and Machine Learning in Performance Marketing | Media Culture – https://www.mediaculture.com/insights/leveraging-ai-and-machine-learning-in-performance-marketing
- AI And Machine Learning In PPC: How To Automate For Maximum Results – https://www.forbes.com/councils/forbesagencycouncil/2024/10/23/ai-and-machine-learning-in-ppc-how-to-automate-for-maximum-results/
- Impact Of Machine Learning On Marketing Analytics – https://www.cometly.com/post/the-impact-of-machine-learning-on-marketing-analytics
- Leveraging AI for Digital Marketing Success – University of Arizona – https://advance.ce.arizona.edu/blog/leveraging-ai-for-digital-marketing-success/
- Applications of Machine Learning in Marketing in 2025 – https://www.analyticsvidhya.com/blog/2023/03/applications-of-machine-learning-in-marketing/
- Machine learning in Marketing – GeeksforGeeks – https://www.geeksforgeeks.org/machine-learning-in-marketing/