Imagine a world where every interaction feels made just for you. That’s what AI-driven customer insights can bring to businesses. It turns data into strategies that meet customer needs. This way, AI makes complex things simple.
Companies like Amazon and Sephora use AI to connect with users. Amazon’s AI-driven recommendations account for 35% of their sales. This shows how important personalization is. Yet, many businesses struggle to use AI to its full power. This article will show how AI changes how we understand customers, helping brands make quicker and smarter decisions.
Key Takeaways
- AI-driven customer insights enable hyper-personalized experiences, boosting satisfaction and loyalty.
- Tools like Zendesk’s AI chatbots reduce wait times while handling queries 24/7, improving support efficiency.
- Predictive analytics identifies trends early, helping businesses innovate before competitors.
- Brands using AI, such as Starbucks, optimize operations and customer engagement through data-driven decisions.
- Adopting AI requires addressing challenges like data overload and bias to maximize benefits.
Understanding AI-Driven Customer Insights
AI-driven customer insights are changing how businesses understand and respond to customer behavior. These systems use algorithms to find patterns in huge datasets that old methods can’t see. For example, customer data analytics tools like sentiment analysis and predictive modeling show what customers like and dislike right away.
What Are AI-Driven Customer Insights?
These insights use machine learning, natural language processing, and predictive analytics to understand customer interactions. For instance, SmartPass uses AI to analyze feedback and make products better. Unlike manual analysis, AI systems quickly sort through millions of data points, like chat logs and social media, to find important trends.
- Machine learning spots changes in buying patterns
- NLP reads feelings from customer reviews
- Predictive analytics predicts demand trends
The Importance of Customer Insights in Business
Business survival depends on using AI-driven customer insights. Over 80% of companies now use generative AI for customer service (McKinsey), and 79% of service professionals say AI is key to their strategy. For example:
Company | Outcome |
---|---|
European Bank | 20% chatbot efficiency gain |
Censornet | 30% rise in feature usage via AI insights |
Starbucks | Personalized offers through behavioral data |
Without customer data analytics, businesses risk being left behind. The consequences are clear: 66% of customers leave brands that don’t personalize. Companies like Amazon and Spotify already use AI to tailor experiences to individual tastes, showing the power of AI-driven strategies.
How AI Enhances Customer Data Analysis
AI changes customer data analytics by making complex tasks easier and finding trends we didn’t see before. It works faster than old methods, giving businesses quick and accurate insights. This helps them make better decisions faster than ever.
Machine Learning and Customer Behavior
Machine learning looks at how customers buy, browse, and interact. It groups customers into segments and predicts their future actions. It also shows how things like price or promotions affect their choices.
- Netflix’s recommendation engine uses machine learning to suggest content, boosting retention by 75%.
- Spotify’s AI DJ analyzes listening history to create personalized playlists in seconds.
Automation in Data Collection
Tools that automate data collection get info from websites, social media, and IoT devices. They save us from doing it all by hand. AI also understands unstructured data, like reviews or chat logs, without bias. For example:
- Brand24 monitors 25 million online sources daily, categorizing sentiment instantly.
- Amazon’s AI updates product recommendations in real time, adapting to shopping cart changes.
By combining machine learning for customer behavior with automation, companies can analyze data 90% faster and more accurately. This means they can turn raw data into useful strategies, making sure they don’t miss any important insights.
The Role of Customer Segmentation
Today, advanced analytics for customer segmentation helps find detailed customer groups. Old ways like age or location are no longer enough. Now, machine learning sorts through huge amounts of data to find hidden patterns. For instance, we used special algorithms for a big retailer, making their strategies more effective by focusing on what each customer likes.
Identifying Key Customer Segments
Good segmentation means looking at many types of data. Here are the main ones:
Data Type | Examples |
---|---|
Demographic | Age, income, geographic region |
Behavioral | Purchase frequency, website interactions |
Transactional | Average spend, product categories used |
Psychographic | Lifestyle choices, brand loyalty indicators |
These details help create useful segments. Tools like CRM and social media analytics give us the data we need.
Personalized Marketing Strategies
Segmentation leads to very focused marketing. Retailers using AI send emails that are 30% more likely to be opened. They match offers to what customers have done before. Our work with a fashion brand saw a 22% increase in sales by matching products to what customers like.
Using these insights, we can create a data-driven customer experience. Teams can send offers to customers who are likely to leave or buy more. This makes customers feel valued and builds loyalty.
Real-Time Insights and Decision Making
AI-driven customer insights help businesses make quick decisions. They can adjust strategies fast because of live data. This is much faster than waiting for delayed analytics.
Benefits of Real-Time Data
Real-time data helps solve problems quickly. It spots issues like support problems or disengagement fast. This can lower customer loss risks by up to 20% in telecom.
CallMiner and NICE inContact cut down manual review time by 40%. They analyze customer interactions right away.
70% of consumers want instant personalization, and real-time systems give it to them.
- Operational agility: 54% of businesses solve issues faster and see 30% higher engagement
- Cost savings: Saves 60% on manual data entry, freeing teams for strategic work
- Predictive action: Finds at-risk accounts 72 hours before old methods do
Case Studies Across Industries
Telecom leaders like Verizon cut churn by 20% with real-time call analysis. Banks like Citibank spot fraud 50% quicker with live monitoring. Retail giants like Walmart boost conversions by 15% with tailored offers.
Healthcare providers like Mayo Clinic see a 60% jump in patient satisfaction with instant feedback. These examples show real-time AI insights bring big wins. As customers expect instant responses, businesses must keep up with these tools. Machine learning keeps improving these systems, meeting changing customer needs.
Predictive Analytics in Customer Insights
Predictive analytics turns raw data into future strategies. It uses past trends to guess future behaviors. This way, businesses can prepare before trends start.
AI-powered customer intelligence solutions now make decisions that were once based on guesses.
What Is Predictive Analytics?
Predictive analytics uses algorithms on past data to predict future outcomes. It looks for hidden patterns in data. This includes analyzing social media posts.
These models give chances of events like customer leaving or buying. They turn guesses into real plans.
Applications in Retail and E-commerce
Retailers and online stores use these tools to increase profits. Here’s how:
- Churn Prediction: Models spot customers at risk, helping keep them. This can reduce churn by 20%.
- Dynamic Pricing: Companies like Amazon change prices quickly. This balances supply and demand.
- Inventory Optimization: Retailers like Target use analytics to stock right items. This cuts overstock by 40%.
- Sentiment Analysis: It analyzes reviews and social media. This helps fix issues before they get worse.
Keeping data and models up-to-date is key. For example, updating customer intelligence monthly keeps predictions current. This has raised customer satisfaction by up to 30% in some cases.
Overcoming Common Challenges
Using AI for customer insights comes with data privacy and system integration challenges. Here’s how to tackle these issues.
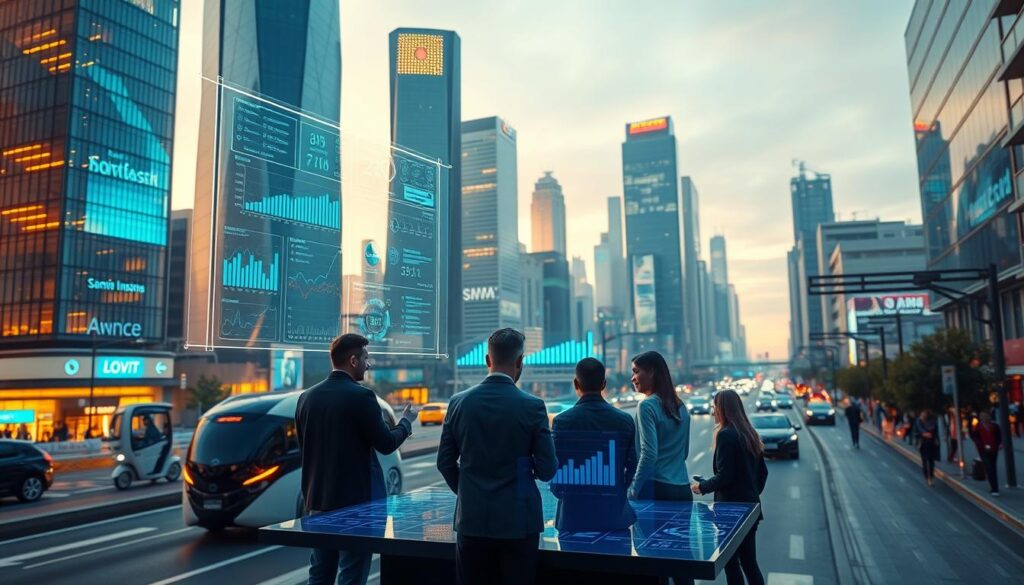
Navigating Data Privacy Safeguards
Following GDPR and CCPA means strict data protection. Use encryption, anonymization, and audits to keep trust. Focus on customer data analytics that respects user consent and offers useful insights.
- Encrypt sensitive data at rest and in transit
- Conduct annual privacy impact assessments
- Implement opt-in mechanisms for data usage
“The #1 barrier to AI adoption is quantifying its value,” states Gartner, highlighting the need for clear metrics in aligning privacy with business goals.
Seamless Integration Strategies
Old systems can’t easily work with new AI tools. To fix this:
- Use APIs to connect CRM platforms with AI engines
- Deploy middleware for real-time data synchronization
- Adopt unified platforms that consolidate customer touchpoints
A credit union found 200 use cases by linking AI with current workflows. Training teams on AI tools cuts agent turnover by 30%, studies show. Choose solutions that boost the data-driven customer experience without changing the core system.
Selecting the Right AI Tools
Choosing the right customer intelligence solutions means picking tools that match your business goals and technical needs. AI has changed how we analyze data. Platforms like Userpilot, Hotjar, and Mixpanel are great at making data useful for strategy. Let’s look at how to pick the best tools.
Popular Tools for Customer Insights
- Userpilot: Combines in-app feedback and behavioral tracking to optimize user journeys.
- Hotjar: Heatmaps and session recordings reveal user behavior patterns instantly.
- Mixpanel: Tracks SaaS metrics and user retention with predictive analytics.
- Zendesk: Integrates support data with CRM systems for unified customer views.
Key Features to Prioritize
Good artificial intelligence in consumer insights tools should:
- Grow with your business and handle big datasets.
- Integrate easily with systems you already use, like CRM or analytics.
- Follow GDPR and CCPA rules for handling user data.
- Have real-time dashboards and reports you can customize for quick decisions.
Tools like Brandwatch and Crayon are top for competitive analysis. SurveyMonkey Genius makes feedback analysis easy. Choose tools that are easy to use but also flexible to avoid setup problems.
Case Studies of Effective Implementation
Businesses all over the world are finding value with AI-driven customer insights and predictive analytics for customer trends. Real examples show how these tools lead to big improvements in different fields.
Success Stories Across Industries
- Spotify’s “Discover Weekly” playlist, powered by predictive analytics for customer trends, now gets over 2.3 billion streams every month. It guesses what music listeners will like.
- Starbucks’ AI-driven app made people more engaged by 20%. It gave personalized offers to get people to come back more.
- BMW cut vehicle downtime by 30% with predictive maintenance. It spots problems before they affect customers.
- JetBlue’s Customer 360 project lowered costs while making customers happier. It paid off in two years.
- Carrefour’s AI chatbot, Hopla, made more people interact online. It also helped make supply chain decisions with quick data insights.
“Our AI systems now spot service trends quicker than ever. This lets us act before customers even notice a problem.” — Telenor spokesperson on Telmi’s implementation
Lessons Learned from AI Implementation
Important lessons from these projects are:
- Working together between tech teams and business leaders is key to aligning goals
- Checking data quality is vital for accurate AI-driven customer insights
- Starting small to test strategies before going big
- Keeping an eye on things to adjust to changing customer habits
These examples show that predictive analytics for customer trends lead to real results with the right plan. Companies like Netflix, who say 80% of views come from AI suggestions, show how these tools can turn data into a strong advantage.
The Future of AI-Driven Customer Insights
Artificial intelligence in consumer insights is growing fast. By 2030, the AI market could hit $1.8 trillion. This change will deeply affect how companies analyze data and connect with customers. Tools like Usersnap are already showing AI’s power, but the future promises even more.
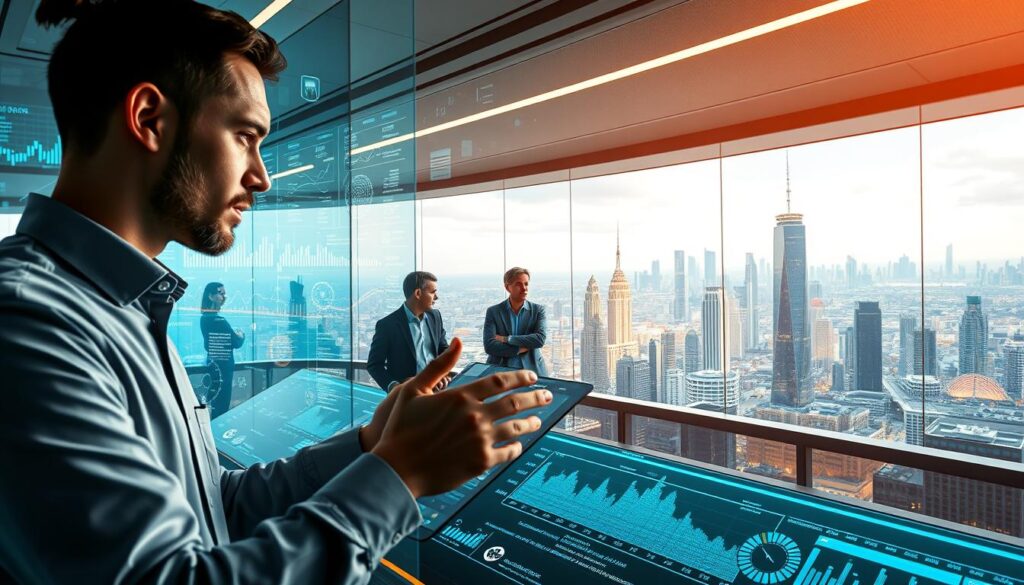
“AI-driven customer insights are transforming how businesses understand and engage with their customers.”
Trends Shaping the Future Landscape
New tech will change how we do advanced analytics for customer groups. Federated learning and generative AI make personal experiences possible without sharing too much data. Emotion AI can spot feelings in real time, and explainable AI makes decisions clear. Here’s a quick look:
Trend | Impact |
---|---|
Federated Learning | Data analysis without centralized storage |
Generative AI | Personalized content at scale |
Emotion AI | Real-time sentiment analysis |
Preparing for Customer Expectations
Customers want fast, personal interactions now. Tools like Salesforce Einstein use predictive analytics for marketing. But, the future needs quick adaptability. Businesses should:
- Use dynamic pricing to match demand, like Amazon’s systems
- Invest in explainable AI to gain trust
- Train teams to mix human intuition with AI
With 68% preferring AI chatbots for quick help, companies must use AI ethically. Platforms like ZenJump show the importance of using AI wisely. By adapting to these changes, businesses can lead in a fast-changing market.
Building a Customer-Centric Culture
Starting with AI tools is just the beginning. To really use customer data analytics well, businesses need a culture that puts the customer first. When teams work together towards common goals, AI insights can lead to real improvements in the data-driven customer experience.
Breaking down silos starts with cross-department collaboration. Marketing, sales, and support teams should all have access to customer data analytics in real-time. This way, they can align their strategies better. For example, 80% of businesses see better satisfaction scores when teams use unified insights.
Regular data reviews and shared dashboards help keep everyone focused on what customers need. Here are some tips:
- Hold weekly cross-functional meetings to discuss customer trends.
- Use centralized platforms to track metrics like satisfaction and retention.
- Assign customer advocacy roles to ensure every team prioritizes user feedback.
Teams need training to understand AI outputs and apply insights. Over 55% of firms use generative AI for personalization. But, success depends on knowing how to use tools like sentiment analysis and predictive models. Training should cover:nnExecutive education on AI’s role in decision-making.nHands-on sessions for analysts to use tools like Tableau or Salesforce Einstein.nWorkshops on ethical use of customer data to maintain trust.nnn63% of service teams say AI helps resolve issues faster, but only if staff are trained to use it effectively (Salesforce survey).n
By combining technical skills with a focus on the customer, organizations can turn data into strategies that increase loyalty and growth.
Ethical Considerations in AI Usage
As artificial intelligence in consumer insights grows, ethical issues need quick action. Companies using customer intelligence solutions must focus on fairness, openness, and trust. Studies reveal over 180 cognitive biases can affect AI results, leading to unfair outcomes. Find out how to make technology align with ethics to safeguard business integrity and customer trust.
Ensuring Fairness and Transparency
Creating unbiased artificial intelligence in consumer insights requires effort:
- Use diverse training data to avoid skewed outcomes
- Conduct regular bias audits for algorithms
- Disclose data usage practices clearly to customers
Balancing Profit and Customer Trust
Success in business depends on ethical AI use. Here are key steps:
Practice | Impact |
---|---|
Opt-in data collection | Builds trust through consent |
Human oversight in AI decisions | Mitigates algorithmic errors |
GDPR/CCPA compliance | Avoids legal risks |
Guidelines like the OECD AI Principles help with ethical AI. By adding fairness checks to customer intelligence solutions, businesses can turn ethics into a competitive edge. Transparency leads to lasting loyalty, showing that ethical AI is not just about following rules—it’s a way to grow.
Conclusion: Embracing AI for Better Customer Relationships
As markets change, businesses must keep up. AI-driven insights are now key to success. By using customer intelligence solutions, companies get real-time data and trends. This turns data into plans that work.
Machine learning helps find patterns in customer behavior. This ensures every interaction meets their needs.
Summary of Key Takeaways
AI changes how businesses talk to customers. Tools like real-time sentiment analysis and machine learning for customer behavior replace old methods. They give insights that help improve customer loyalty.
These solutions cut down on delays and give accurate data. This leads to better retention and growth. Plus, using AI ethically builds trust with customers.
Encouragement to Start Implementing AI-Driven Insights
Start with easy tools like free analytics or AI modules. Begin with small tests to see how well they work. Look for customer intelligence solutions that fit with what you already use.
Working with experts can help overcome challenges. Even small steps, like analyzing social media or improving email campaigns, can make a big difference.
At Curamando, we’ve seen clients increase NPS scores by 30% with real-time analytics. Don’t wait; the gap between those who use AI and those who don’t grows every day. Check out our resources, attend workshops, or ask for a demo to begin. The future of customer relationships is AI-powered, and it begins now.
FAQ
What are AI-driven customer insights?
Why are customer insights important for businesses?
How does machine learning enhance customer behavior analysis?
What role does automation play in data collection?
How can businesses effectively segment their customers?
What are the benefits of real-time insights in a business environment?
What is predictive analytics in customer insights?
What common challenges do organizations face when implementing AI-driven customer analytics?
How do I choose the right AI tools for customer insights?
Can you provide examples of successful AI-driven customer insights implementations?
What are the emerging trends in AI-driven customer insights?
How can organizations build a customer-centric culture for AI utilization?
What ethical considerations should businesses keep in mind when using AI in customer insights?
Source Links
- How to Leverage Customer Insights AI to Grow Your SaaS Product – https://userpilot.com/blog/customer-insights-ai/
- Revolutionizing Retail with AI-Driven Customer Insights – https://www.netguru.com/blog/revolutionizing-retail-with-ai-driven-customer-insights
- How AI Can Unlock Customer Insights [+Expert Tips] – https://blog.hubspot.com/service/customer-insights-ai
- VoC and AI: Revolutionizing Customer Insights – https://www.cmswire.com/customer-experience/unlocking-the-voice-of-customer-with-ai/
- Leveraging AI in Dynamics 365 Customer Insights and Journeys – https://www.alphabold.com/leveraging-ai-in-dynamics-365-customer-insights-and-journeys/
- “Unlocking Customer Insights Through AI-Driven Data Analysis” – https://medium.com/@jesse.henson/unlocking-customer-insights-through-ai-driven-data-analysis-ff58b6498e0e
- Your Complete Guide To AI and Customer Data – https://tealium.com/blog/artificial-intelligence-ai/your-complete-guide-to-ai-and-customer-data/
- AI customer insights: The AI advantage in consumer research – https://www.zappi.io/web/blog/ai-customer-insights-the-ai-advantage-in-consumer-research/
- AI-driven customer segmentation: A step-by-step guide – https://www.revenuemarketingalliance.com/ai-driven-customer-segmentation/
- AI Customer Segmentation Strategies | Mailchimp – https://mailchimp.com/resources/ai-customer-segmentation/
- The Evolution of Customer Success Relies on Real-Time Insights | Gainsight Software – https://www.gainsight.com/blog/the-evolution-of-customer-success-relies-on-real-time-insights/
- What are Real-time Customer Insights? | NICE – https://www.nice.com/glossary/real-time-customer-insights
- How AI Improves Real-Time Strategic Decision-Making in Call Centers – Insight7 – AI Tool For Interview Analysis & Market Research – https://insight7.io/how-ai-improves-real-time-strategic-decision-making-in-call-centers/
- Create and manage predictions – Dynamics 365 Customer Insights – https://learn.microsoft.com/en-us/dynamics365/customer-insights/data/predictions
- Predictive Customer Insights Using AI for Better Service Delivery 1 – https://callcenterstudio.com/blog/predictive-customer-insights-using-ai-for-better-service-delivery/
- Overcoming Common Customer Experience Challenges – https://www.cdw.com/content/cdw/en/articles/digitalworkspace/overcoming-common-customer-experience-challenges.html
- How to Overcome the 5 Biggest Challenges in AI Implementation – https://www.linkedin.com/pulse/how-overcome-5-biggest-challenges-ai-implementation-b-eye-ltd-axhef
- 15 AI Market Research Tools For Smarter Consumer Insights – https://www.gwi.com/blog/ai-market-research-tools
- AI for Customer Insights | 12 Tools for 2025 – https://www.crescendo.ai/blog/ai-for-customer-insights-tools
- How to choose the right AI solution for insights-driven business decisions | Market Logic – https://marketlogicsoftware.com/blog/how-to-choose-the-right-ai-solution-for-insights-driven-business-decisions/
- Successful Customer Experience (CX) with AI Implementation – https://www.renascence.io/journal/case-studies-successful-customer-experience-cx-with-ai-implementation
- Case Studies: Successful Implementation of Generative AI Services – https://www.linkedin.com/pulse/case-studies-successful-implementation-generative-ai-services-0ifvc
- Case Studies: Success Stories of AI in Customer Experience – https://www.linkedin.com/pulse/case-studies-success-stories-ai-customer-experience-nicolas-babin-zmyqe
- The Future of Marketing Analytics: AI-Driven Insights – https://www.and-marketing.com/future-of-ai-in-marketing-analytics/
- 7 Ways Customer Experience and AI Will Evolve in 2024 – https://www.superoffice.com/blog/customer-experience-ai/
- Attract to amaze: The future of customer experience in an AI-driven world – https://www.pwc.com/us/en/services/consulting/business-transformation/library/rise-of-ai-customer-experience.html
- Customer Service: How AI Is Transforming Interactions – https://www.forbes.com/councils/forbesbusinesscouncil/2024/08/22/customer-service-how-ai-is-transforming-interactions/
- Ethical Considerations in AI-Driven Customer Segmentation – https://www.usaii.org/ai-insights/ethical-considerations-in-ai-driven-customer-segmentation
- Ethical Considerations in AI-Driven Decision Making – https://www.linkedin.com/pulse/ethical-considerations-ai-driven-decision-making-nicole-hein-zvy6c
- The 3 Pillars of Search Engine Optimization – https://www.nobledesktop.com/learn/digital-marketing/the-impact-of-ai-on-customer-relationship-management
- Beyond NPS: Embracing AI-Driven Customer Satisfaction Metrics – https://www.linkedin.com/pulse/beyond-nps-embracing-ai-driven-customer-satisfaction-metrics-lewis-m8kve