Every marketer has faced the quiet loss of a customer who simply disappears—no complaint, no warning. What if you could predict that silence and turn it into an opportunity? That’s where predictive analytics in marketing steps in. Today’s leading brands, like ZlavaDna and Missguided, are using data-driven marketing to anticipate needs, personalize offers, and drive results. As Deepak Bansal emphasizes, “Leveraging data-driven insights can significantly enhance marketing strategies,” and this guide reveals how.
Imagine knowing when a customer is likely to leave before they do—or boosting email open rates by 400% by targeting the right audience at the right time. These aren’t guesses; they’re proven outcomes from brands using marketing analytics to turn data into action. From reducing churn to maximizing revenue, predictive analytics isn’t just a tool—it’s the compass guiding today’s most successful campaigns.
Key Takeaways
- Predictive analytics improves conversion rates by up to 10% through personalized experiences.
- E-commerce sees 20% higher average order values via data-driven product recommendations.
- Data-driven strategies achieve 5-8x higher ROI compared to traditional methods.
- Unified data integration boosts marketing effectiveness by 25% by unifying customer insights.
- Predictive marketers are 2.9 times more likely to outperform industry revenue averages.
Behind every statistic is a story of businesses that stopped guessing and started predicting. Let’s explore how you can harness these strategies to transform your campaigns, starting today.
Understanding Predictive Analytics in Marketing
Predictive analytics in marketing uses old data and smart algorithms to guess what will happen next. It helps companies make smart choices by looking at how people act and buy things. This is all thanks to predictive modeling, which predicts what might happen based on past data.
Let’s look at how it has changed. At first, it was simple, but now it uses AI and machine learning. Here’s a quick timeline of major improvements:
Year | Development |
---|---|
1980s | Introduction of basic statistical modeling for sales forecasting |
2000s | Rise of CRM systems integrating customer data for segmentation |
2010s | AI and neural networks enable real-time predictive modeling |
Now, predictive analytics in marketing is a must-have. More than 70% of companies say it helps them understand their customers better. For example, telecoms cut down on lost customers by 5-10% by analyzing how they use their services. Retailers also save 20% on inventory by predicting demand.
Companies that use this approach grow 20% faster than others. It’s a big win.
“Predictive analytics transforms raw data into strategic foresight, turning guesswork into actionable plans.”
As the market gets more crowded, predictive analytics helps brands stay ahead. It helps them spend their ad budget wisely, tailor offers to customers, and spot trends early. With data-driven marketing, every choice is based on facts, not just guesses.
The Components of Predictive Analytics
Data analytics in marketing is a systematic approach to interpreting data for strategic decisions, enabling agile business operations through insights.
Modern marketing analytics has three main parts: data collection, machine learning algorithms, and visualization tools. These elements are key to predictive modeling. They turn raw data into strategies that can be acted upon. Over 59.5% of businesses use these tools to innovate, as shown by 2023 research by NewVantage Partners. Let’s look at each part:
Component | Description | Marketing Impact |
---|---|---|
Data Collection | Aggregating data from CRM systems, transactions, and social media | Enables accurate predictive modeling |
Machine Learning | Algorithms like decision trees and neural networks | Automates pattern detection for marketing decisions |
Visualization Tools | Software like Tableau or Power BI | Makes insights accessible to non-technical teams |
Data Collection and Management
Quality data is essential. Companies gather data from CRM systems, website analytics, and surveys. Data governance frameworks help keep data accurate—91.9% of companies saw ROI from these practices. Clean data ensures predictive models are reliable and ethical.
Machine Learning Algorithms
Machine learning in marketing uses techniques like regression analysis to predict customer behavior. Neural networks find hidden patterns, and decision trees simplify decisions. These tools help target campaigns more effectively.
Data Visualization Tools
Tools like Tableau make complex data easy to understand. Teams can track trends quickly, keeping strategies up-to-date. Clear visuals improve teamwork and reduce delays in decision-making.
Understanding these components helps teams predict outcomes, manage budgets, and beat competitors. Learn more about data governance here.
Benefits of Predictive Analytics for Businesses
Predictive analytics takes data analysis a step further by forecasting future trends and behaviors.
Predictive analytics turns raw data into strategic advantages. It predicts trends, reducing uncertainty and driving growth. These insights create measurable value across industries.
Enhanced Customer Insights
Understanding predictive customer behavior unlocks opportunities to tailor experiences. Retailers like Amazon use purchase histories to suggest products, boosting sales. By analyzing interactions, companies can identify unmet needs and design solutions before competitors.
This foresight ensures products and services align with evolving preferences.
Improved ROI on Marketing Campaigns
Marketing analytics sharpens campaign precision. Targeted messaging reduces ad waste while boosting conversions. Walmart cut inventory costs by 15% through predictive demand forecasts.
Capital One reduced loan defaults by 25% via risk modeling. Such digital marketing strategy optimizations turn data into tangible gains.
Streamlined Marketing Strategies
Predictive tools eliminate guesswork in planning. Allstate improved insurance pricing accuracy by 20%, and delivery firms slashed fuel costs by 15% through route optimization. These streamlined workflows reduce redundancies, freeing resources for innovation.
Industry | Company | Outcome |
---|---|---|
Retail | Amazon | 35% sales rise via product recommendations |
Banking | Capital One | 25% improvement in credit risk assessments |
Insurance | Allstate | 20% more accurate pricing |
Delivery | Delivery Companies | 15% fuel cost reduction |
Key Data Sources for Predictive Analytics
Data-driven marketing relies on good data. Let’s look at the main sources for predictive analytics in marketing:
- CRM platforms like Salesforce or HubSpot track purchase histories and service interactions.
- Target’s predictive modeling analyzed CRM data to identify pregnant customers through product purchases, boosting retention through personalized offers.
- Customer segmentation becomes precise when CRM data reveals buying patterns and preferences.
Social Media & Digital Activity:
- Platforms like Facebook and Google Analytics monitor online behavior, revealing sentiment trends and content preferences.
- Adobe Analytics uses this data to predict churn risks, enabling real-time adjustments to campaigns.
- Data from social media fuels data-driven marketing by highlighting emerging trends before they go viral.
Transactional Records:
- Sales data uncovers high-value customer segments and seasonal buying cycles.
- Businesses using this data report up to 20% higher conversion rates and 25% improved gross margins.
- Tools like Microsoft Azure Machine Learning analyze transactional data to forecast demand and optimize inventory.
By combining these sources, we can map the full customer journey. Using CRM, digital behavior, and sales metrics ensures predictive models reflect real-world consumer actions. For example, Starbucks uses transactional data to tailor loyalty rewards, driving repeat visits. Prioritizing data quality and privacy compliance unlocks the full power of predictive analytics in marketing.
Implementing Predictive Analytics Strategies
The intersection of behavior and analytics opens up exciting possibilities for marketers to dive deeper into consumer psychology.
To start using predictive analytics, you need a solid plan. First, make sure your predictive modeling fits with your digital marketing strategy. Here’s a step-by-step guide to help you succeed:
Step-by-Step Guide
- Define Objectives: Know what you want to achieve, like keeping customers or making more from campaigns.
- Gather Data: Collect all kinds of data to analyze, like CRM and transaction records.
- Build Models: Use machine learning in marketing to predict how customers will behave.
- Test & Iterate: Check how well your models work and keep improving them.
- Integrate Insights: Use what you learn to make decisions right away.
Common Tools and Software
Tool | Key Features |
---|---|
Adobe Analytics | Predictive segmentation and campaign optimization |
Google Analytics 360 | Advanced attribution modeling and customer journey tracking |
IBM Watson Studio | Automated machine learning for predictive modeling |
Building a Cross-Functional Team
For success, you need a team that mixes digital marketing strategy know-how with data science skills. Important roles include:
- Data Engineers: Make sure the data is clean and ready.
- Analysts: Work on and test the predictive models.
- Marketers: Use the insights to improve campaigns and content.
Smaller teams can work with outside agencies or train staff in Python or R programming.
Real-World Applications in Marketing
Predictive analytics turns raw data into real plans. Let’s see how companies use these tools to get results.
- Airbnb used predictive analytics to improve their digital marketing strategy. They grew by 43,000% in just five years.
- A big food retailer increased coupon use by 15%. They analyzed predictive customer behavior and ran more targeted campaigns in 10,000+ stores.
- Würth used marketing analytics to sort customers into groups with 85% accuracy. This helped them spend their marketing budget better and engage more.
Success stories are found across different industries. Retail, hospitality, and B2B all use predictive analytics in their own ways. For instance, e-commerce sites use it to match inventory with seasonal demand. They also personalize websites for each visitor in real-time.
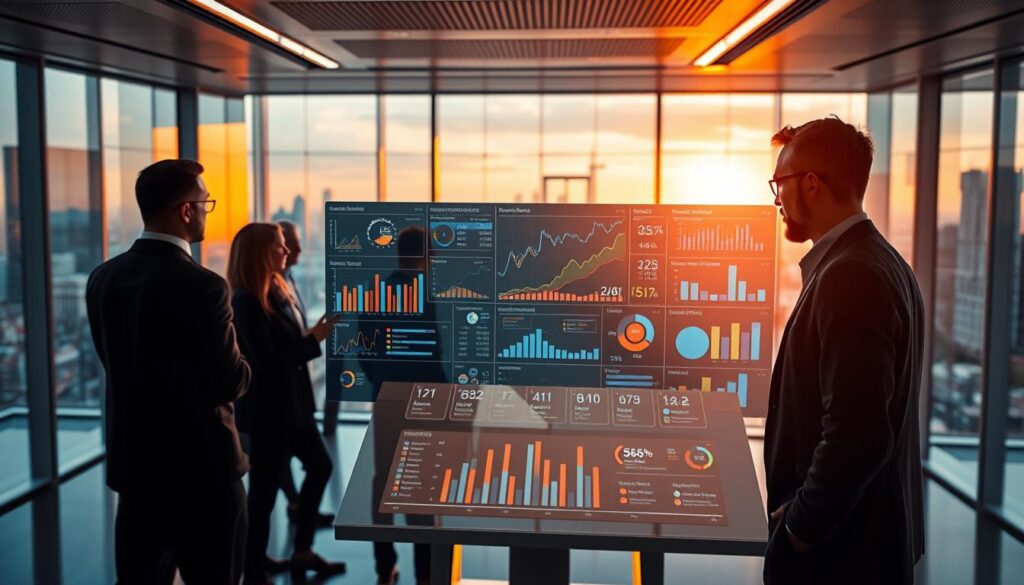
New uses of predictive analytics are always emerging. One company cut fraud detection costs by 72% with predictive models. Others use AI to test creative ads. As one expert said:
AI-powered analytics uncovers insights human teams miss, enabling strategies that truly resonate.
These stories show predictive analytics is more than just theory. It’s a tool any business can use. It helps improve marketing campaigns and guess what customers want. This turns data into a strong advantage in the market.
Overcoming Challenges in Predictive Analytics
Using predictive analytics in marketing can change the game, but it’s not without its challenges. Issues like data privacy, system compatibility, and finding the right talent can hold you back. To make the most of it, you need to tackle these problems head-on. Studies show that 60% of businesses struggle with privacy, and 70% face integration problems. Here’s how to overcome these hurdles.
Data Privacy Concerns
Keeping customer data safe is a must. You need to follow GDPR and CCPA rules, which mean getting clear consent and using anonymization. Using privacy-by-design helps make your data-driven marketing strategies ethical. Regular checks and encryption keep your data safe while keeping your analysis accurate.
Integrating with Existing Systems
Old systems can be hard to mix with new ones. To make it work, focus on APIs and middleware for smooth data flow. Choose platforms that handle real-time data well. Studies show that using unified analytics platforms can cut integration time by 30%. Here’s what to do:
- Check your current tech to see what works together
- Start small with integration in non-critical areas
- Go for cloud-based solutions for easy growth
Skills Gap in Analysis and Interpretation
The need for analytics skills will jump 28% by 2030. To fill this gap:
- Teach your team about tools like Python and Tableau with workshops
- Work with agencies that know marketing analytics for tough tasks
- Choose platforms that are easy to use and deploy
Keep learning and work together across departments to get better at using analytics. Every small step today helps build a strong base for tomorrow.
The Role of AI in Predictive Analytics
AI changes predictive analytics by combining machine learning in marketing with live data. It’s different from old methods that can’t keep up with changing trends. For example, Deloitte says AI boosts forecasting by 30%. McKinsey notes a 20% drop in inventory costs with AI.
There are two main ways AI helps: augmented decision-making and autonomous systems. IBM’s quantum computing could cut analysis time by 80%. Gartner says AI makes marketing 40% more efficient by automating data work.
- Machine Learning vs. Traditional Analytics: AI finds patterns in data that older methods miss, like what customers buy or post online.
- Automation in Action: AI tools now handle ad targeting, budgeting, and personalizing content without human help.
- Future Frontiers: Trends like NLP for understanding feelings and computer vision for improving visuals will be big in 2024, MIT Sloan predicts.
AI’s power is in making data useful, but we must watch for bias.
Forrester found AI can reduce errors by 28% when used right. By 2024, 50% of companies will use deep learning, MIT says. This will change how machine learning in marketing connects with customers.
Crafting Personalized Marketing Campaigns
Effective customer segmentation begins with understanding how people behave and what they might do next. By dividing audiences into smaller groups, brands can send messages that really speak to them. For example, Coca-Cola’s “Share a Coke” campaign used names to connect with people, showing how personal touches can make a big difference.
“Personalization can improve consumer satisfaction, brand loyalty, engagement, and conversion rates.” — McKinsey Report
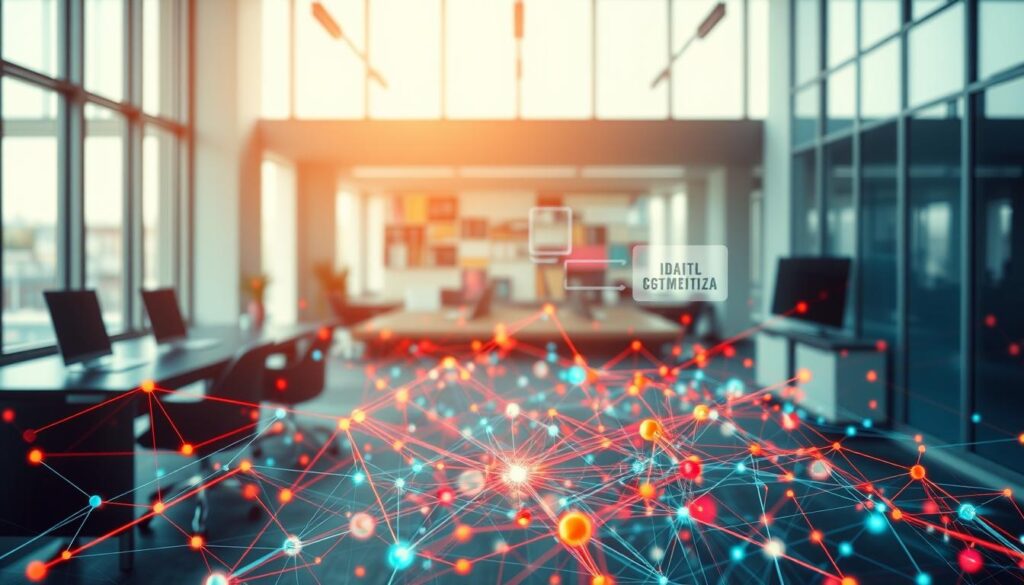
When you mix customer segmentation with a smart digital marketing strategy, you get campaigns that really work. Tools like Google Analytics and CRM systems help track how people interact with your brand. For instance, Spotify’s Year in Music emails use what you’ve listened to to make a special summary, which gets more people to open them by 29%.
- Use A/B testing to refine messaging for different segments
- Align offers with predicted lifecycle stages (e.g., post-purchase follow-ups)
- Monitor metrics like click-through rates to gauge relevance
To see if your campaign is working, you need to watch things like how many people buy and how much they spend over time. Brands like Sephora use smart data to suggest products, which can increase what people spend by 20%. But it’s also important to respect people’s privacy. Following rules like GDPR helps keep trust while making sure your campaigns are effective. By using data wisely and being ethical, you can make campaigns that feel like they were made just for you, not like they’re trying to get too much.
The Importance of Data Quality
Every day, 2.5 quintillion bytes of data are created, but not all is useful. For predictive modeling and data-driven marketing, high-quality data is essential. Without it, even the most advanced marketing analytics tools fail.
“The foundation of effective data analytics lies in the quality and accuracy of the data utilized.” – Industry Data
Ensuring Accurate Predictions
Bad data can ruin predictive modeling. For example, duplicate customer records can mess up churn predictions. Old purchase history can also lead to wrong campaign timing. Big names like Amazon and Starbucks clean their data well to avoid these problems. Some common issues include:
- Inconsistent entries: Confusing “NYC” and “New York” in CRM systems.
- Missing fields: Gaps in demographics reduce segmentation accuracy.
- Outdated info: Old contact details hinder targeted outreach.
Best Practices in Data Management
Building a strong framework starts with:
- Automated audits: Tools like Talend scan datasets weekly.
- Standardized formats
- Cross-departmental reviews: Marketing and IT teams collaborate to flag anomalies.
Tools for Data Quality Assessment
Tool | Purpose | Use Case |
---|---|---|
Microsoft Excel | Data cleansing | Removing duplicates in customer lists |
Alteryx | Automated workflows | Linking CRM and sales data |
Trifacta | Visual anomaly detection | Spotting outliers in social media engagement |
These tools make sure only clean data goes into marketing analytics pipelines. Making data integrity a priority is not just technical—it’s key for smart data-driven marketing strategies.
Measuring Success with Predictive Analytics
It’s key to track results to make sure predictive analytics is worth it. Start by picking the right metrics to check how well it’s doing. Marketing analytics experts say to focus on three main things: how accurate the predictions are, the impact on the business, and how efficient it is.
“By monitoring key performance indicators (KPIs) like click-through rates, conversion rates, and ROI, you can assess campaign effectiveness in real time.”
Key Performance Indicators (KPIs)
To do well with predictive analytics in marketing, you need to watch these KPI categories:
- Prediction Accuracy: Look at how well forecasts match real results (like Hydrant’s 2.6x conversion rate boost)
- Business Impact: Keep an eye on things like how much it costs to get a new customer and how well you keep them
- Operational Efficiency: See how much time and resources you save in planning and running campaigns
Adjusting Strategies
Data-driven marketing teams use insights to:
- Move budgets to channels that work best (like Amazon’s demand forecasting)
- Improve how they segment customers (like Netflix’s algorithm updates)
- Update predictive models with fresh data (Salesforce’s lead scoring improvements)
Long-Term Impact
Category | Example Metrics | Business Outcome |
---|---|---|
Prediction Accuracy | Churn prediction accuracy rates | Reduced customer attrition |
Business Impact | CLV increases | Higher lifetime value |
Operational Efficiency | Resource allocation efficiency | Cost savings |
Checking these metrics often helps stay ahead. Companies like Coca-Cola use this to make campaigns 20% more effective. By linking KPIs to goals, teams make sure predictive models lead to real results over time.
Future Trends in Predictive Analytics
As predictive analytics grows, businesses need to update their digital marketing plans. They must use new innovations like real-time insights and cross-technology integrations. These changes will help them understand customer behavior better, drive growth, and tackle ethical issues.
The Rise of Real-Time Analytics
Real-time analytics platforms do more than track trends; they predict actions. With 5G and edge computing, companies can analyze customer interactions right away. A health brand saw a 40% increase in organic traffic by making quick changes.
These tools help track customer behavior in real-time. They cut response times and boost conversions by up to 30%.
Integration with Other Technologies
Predictive marketing trends now involve combining analytics with new technologies. Healthcare uses AI to predict patient outcomes, and finance uses models to manage stock risks. IoT and blockchain improve data accuracy, and AR/VR create immersive experiences.
Automation platforms like Salesforce Einstein make these tools easy to use. This lowers barriers for teams without technical skills.
Evolving Consumer Behavior and Expectations
Today, consumers want transparency and control over their data. This changes how predictive marketing works. Ethical AI frameworks are needed to balance personalization with privacy.
For example, a nonprofit increased donations by making campaigns more personal but ethical. Quantum computing will make models even better. But success depends on using technology that aligns with changing values.
Businesses must focus on fairness and clarity. They need to use predictive customer behavior insights to build trust.
FAQ
What is predictive analytics in marketing?
How has predictive analytics evolved over time?
Why is data quality important for predictive analytics?
What are the main benefits of using predictive analytics in marketing?
What are some key data sources used in predictive analytics?
How can organizations implement predictive analytics strategies?
What types of challenges do companies face when adopting predictive analytics?
How does AI enhance predictive analytics in marketing?
What is the role of customer segmentation in predictive marketing?
How can the effectiveness of predictive analytics initiatives be measured?
What future trends should marketers watch regarding predictive analytics?
Source Links
- How To Use Data Analytics To Drive Digital Marketing Success – https://www.forbes.com/councils/forbesbusinesscouncil/2024/07/19/how-to-use-data-analytics-to-drive-digital-marketing-success/
- Leveraging Predictive Analytics Techniques for Business Growth – https://www.pecan.ai/blog/leveraging-predictive-analytics-techniques-business/
- Drive Revenue With Predictive Marketing – https://www.bloomreach.com/en/blog/predictive-marketing
- Understanding Predictive Analytics: A Comprehensive Guide – https://blog.trace3.com/understanding-predictive-analytics-a-comprehensive-guide
- The Best Guide to Predictive Analytics in Marketing | 6sense – https://6sense.com/platform/predictive-analytics/predictive-analytics-in-marketing/
- An All-In-One Guide for a Data-Driven Future – https://www.markovml.com/blog/predictive-analysis
- The Role of Predictive Analytics in Marketing – https://www.techfunnel.com/martech/predictive-analytics-marketing-role/
- What Is Predictive Analytics? Benefits, Examples, and More – https://www.coursera.org/articles/predictive-analytics
- What is predictive analytics? Importance, benefits, & examples – https://www.imd.org/blog/digital-transformation/what-is-predictive-analysis/
- Predictive Analytics for Marketing to Maximize Conversions – https://data-sleek.com/blog/enhance-conversions-predictive-analytics-for-marketing/
- Predictive Analytics For Marketing Teams – https://www.cometly.com/post/predictive-analytics-in-marketing
- Domo Resource – Top 8 Predictive Analytics Tools – https://www.domo.com/learn/article/predictive-analytics-tools
- Predictive analytics for marketing: What you need to know | Webflow Blog – https://webflow.com/blog/predictive-analytics-marketing
- How to Implement Predictive Analytics in Market Research – https://www.greenbook.org/insights/research-methodologies/how-to-implement-predictive-analytics-in-market-research
- Predictive Analytics in Marketing – https://www.fourfront.us/blog/how-to-use-predictive-analytics-for-marketing/
- 7 Predictive Analytics Examples To Inspire Your Next Move – https://thecmo.com/digital-marketing/predictive-analytics-examples/
- 10 Powerful Predictive Analytics Examples & Use Cases – https://www.teramind.co/blog/predictive-analytics-examples/
- Why Predictive Analytics Matters: Exploring the Game-Changing Benefits – https://www.pecan.ai/blog/benefits-of-predictive-analytics/
- The Power of Predictive Customer Analytics – https://www.launchnotes.com/blog/the-power-of-predictive-customer-analytics
- Integrating Predictive Analytics with Marketing Automation – https://www.techfunnel.com/martech/predictive-analytics-marketing-automation/
- The Power Of AI In Predictive Analytics – https://swifterm.com/the-power-of-ai-in-predictive-analytics/
- The Role Of AI In Predictive Analytics: How Machine Learning Is Transforming Forecasting – Boston Institute Of Analytics – https://bostoninstituteofanalytics.org/blog/the-role-of-ai-in-predictive-analytics-how-machine-learning-is-transforming-forecasting/
- The Power Of Personalization: Crafting Tailored Marketing Campaigns For Maximum Impact – https://www.forbes.com/councils/forbesbusinesscouncil/2024/07/22/the-power-of-personalization-crafting-tailored-marketing-campaigns-for-maximum-impact/
- Crafting Success: The Art of Tailored Campaigns – https://www.salesgasm.com/post/crafting-success-the-art-of-tailored-campaigns
- The 3 Pillars of Search Engine Optimization – https://www.nobledesktop.com/learn/digital-marketing/ai-and-predictive-analytics-the-future-of-marketing-insights
- SQL for Business Analysts – https://www.nobledesktop.com/learn/data-analytics/the-importance-of-data-quality-in-predictive-analytics
- What is Predictive Analytics in Marketing? (7 Use Cases) – https://jetpack.com/resources/what-is-predictive-analytics-in-marketing/
- The Role of Predictive Analytics in Marketing – https://www.linkedin.com/pulse/role-predictive-analytics-marketing-netsqure-24odc
- Complete Marketing Predictive Analytics Guide | NoGood – https://nogood.io/2024/07/17/marketing-predictive-analytics/
- 3 ways to use predictive analytics to make better decisions | MarTech – https://martech.org/3-ways-to-use-predictive-analytics-to-make-better-decisions/
- Maximize Your Marketing ROI with Predictive Analytics – https://www.cardinalpath.com/blog/maximize-your-marketing-roi-with-predictive-analytics
- The Future of Predictive Analytics: Trends and Innovations to Watch – https://datafloq.com/read/the-future-of-predictive-analytics-trends-and-innovations-to-watch/
- Predictive Analytics In Marketing – AgencyAnalytics – https://agencyanalytics.com/blog/predictive-analytics-in-marketing